Is anonymized data of any value?
Anonymization is still sometimes criticized as a practice that supposedly makes data worthless, as it deletes important information. The CNIL decided to prove the contrary through the Cabanon project conducted in 2017. It received assistance from the IMT big data platform, TeraLab, for anonymizing the data of New York taxis and showing the possibility of creating a transportation service.
On 10 March 2014, an image published on Twitter by the New York taxi commission sparked Chris Whong’s curiosity. It wasn’t the information on the vehicle occupancy rate during rush hour that caught the young urban planner’s interest. Rather, what caught his eye was the source of the data, cited at the bottom, that had allowed New York City’s Taxi and Limousine Commission (NYC TLC) to create the image. Through a tweet comment, he joined another Twitter user, Ben Wellington, in asking if the raw data was available. What ensued was a series of exchanges that enabled Chris Whong to retrieve the dataset through a process that is tedious, yet accessible to anyone with enough determination to cut through all the red tape. Once he had the data in his possession, he put it online. This allowed Vijay Pandurangan, a computer engineer, to demonstrate that the identity of the drivers, customers, and their addresses could all be found using the information stored on the taxi logs.
Problems in anonymizing open datasets are not new. They were not even new in 2014 when the story emerged about NYC TLC data. Yet this type of case still persists. One of the reasons is that anonymized datasets are deemed less useful than their unfiltered counterparts. Removing any possibility of tracing the identity would amount to deleting the information. In the case of the New York taxis, for example, this would mean limiting the information on the taxis’ location to geographical areas, rather than indicating the coordinates to the nearest meter. For service creators who want to build applications, and data managers who want the data to be used as effectively as possible, anonymizing means losing value.
As a fervent advocate for the protection of personal data, the French National Commission for Information Technology and Civil Liberties (CNIL) decided to confront this misconception. The Cabanon project, led by the CNIL laboratory of digital innovation (LINC) in 2017, took on the challenge of anonymizing the NYC TLC dataset and using specific scenarios for creating new services. “There are several ways to anonymize data, but there is no miracle solution that fits every purpose,” warns Vincent Toubiana, in charge of anonymizing the datasets for the project, which has since transferred from the CNIL to the ARCEP. The Cabanon team therefore had to think of a dedicated solution.
Spatial and temporal degradation
First step: the GPS coordinates were replaced by the ZCTA code, the U.S. equivalent of postal codes in France. This is the method chosen by Uber to ensure personal data security. This operation degrades the spatial data; it drowns the taxi’s departure and arrival positions in areas composed of several city blocks. However, this may prove insufficient in truly ensuring the anonymity of the customers and drivers. At certain times during the night, sometimes only one taxi made a trip from one area of the city to another. Even if the GPS positions are erased, it is still possible to link the geographical position and identity.
“Therefore, in addition to the spatial degradation, we had to introduce a temporal degradation,” Vincent Toubiana explains. The time slots are adapted to avoid the single customer problem. “In each departure and arrival area, we look at all the people who take a taxi in time slots of 5, 15, 30 and 60 minutes,” he continues. In the data set, the time calibration is adjusted so that no time slot has fewer than ten people. If, despite these precautions, a single customer is within the largest time slot of 60 minutes, the data is simply deleted. According to Vincent Toubiana, “the goal is to find the best mathematical compromise for keeping a maximum amount of data with the smallest possible time intervals.”
In the 2013 data used by the CNIL, the same data made public by Chris Whong, NYC TLC made over 130 million trips. The double degradation operations therefore demanded significant computing resources. The handling of the data to be processed using different temporal and spatial slicing required assistance from TeraLab, IMT’s big data platform. “It was essential for us to work with TeraLab in order to query the database to see the 5-minute intervals, or to test the minimum number of people we could group together,” Vincent Toubiana explains.
Read more on I’MTech: Teralab, a big data platform with a European vision
Data visualization assisting data usage
Once the dataset has been anonymized in this way, it must be proven useful. To facilitate its reading, a data visualization in the form of a choropleth map was produced—a geographical representation associating a color with each area based on the amount of trips. “The visual offers a better understanding of the differences between anonymized data and that which is not, and facilitates the analysis and narration of this data,” says Estelle Hary, designer at the CNIL who produced the data visualization.
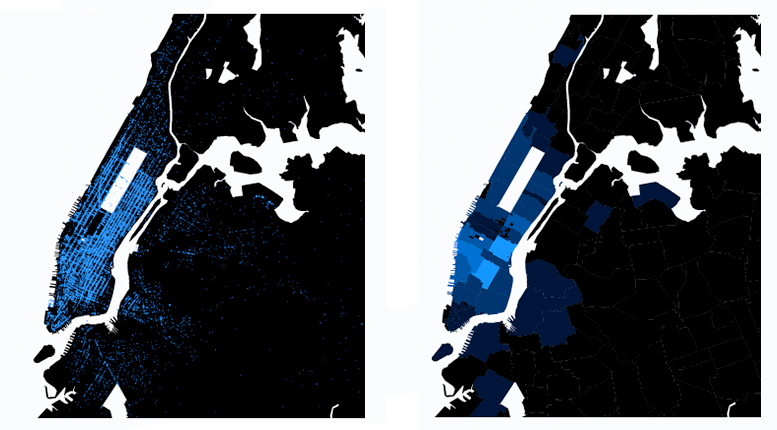
To the left: a map representing the trips using non-anonymized data. To the right: choropleth map representing the journeys with a granularity that ensures anonymity.
Based on this map, they began reflection on the kinds of services that could be created using anonymized data. The map helped identify points in Brooklyn where people order taxis to complete their journey home. “We started thinking about the idea of a private transportation network that would complement public transport in New York,” says Estelle Hary. Since they would be cheaper than taxis, this private public transport could cover areas neglected by buses. “This is a typical example of a viable service that anonymized data can be used to create,” she continues. In this case, the information that was lost to protect the personal data had no impact. The processed data set is just as effective. And this is only one example of a potential use. “By linking anonymized datasets with other public data, the possibilities are multiplied,” the designer explains. In other words, the value of an open dataset depends on our capacity for creativity.
There will certainly always be cases in which the degradation of raw data limits the creation of a service. This is the case for more personalized services. But perhaps anonymity should be seen, not as a binary value, but as a gradient. Instead of seeing anonymity as a characteristic that is present or absent from datasets, wouldn’t it be more appropriate to consider several accessible degrees of anonymity according to the exposure of the data set and the control over the use? That is what is the CNIL proposed in the conclusion of the Cabanon project. The data could be publicly accessible in fully anonymized form. In addition, the same dataset could be accessible in versions that are less and less anonymized, with, in exchange, a more significant level of control over the use.
[box type=”info” align=”” class=”” width=””]
TeraLab, big data service for researchers
Teralab is a big data and artificial intelligence platform serving research, innovation and education. It is led by Institut Mines-Télécom (IMT) and the Group of National Schools of Economics and Statistics (GENES). Teralab was founded in 2014 through a call for projects by the Investments for the Future program called “Cloud Computing and Big Data”. The goal of the platform is to aggregate the demand for software and infrastructure for projects involving large volumes of data. It also offers security and sovereignty, enabling stakeholders to entrust their data to the researchers with confidence. [/box]
Leave a Reply
Want to join the discussion?Feel free to contribute!