When AI helps predict a patient’s care pathway
Researchers at Mines Saint Etienne are using process mining tools to attempt to describe typical care pathways for patients with a given disease. These models can be used to help doctors predict the next steps for treatment or how a disease will progress.
Will doctors soon be able to anticipate patient complications arising from a disease? Will they be able to determine an entire care pathway in advance for patients with a specific disease? These are the goals of Vincent Augusto and his team at Mines Saint-Étienne. “Based on a patient’s treatment records, their condition at a given moment, and care pathways of similar patients, we’re trying to predict what the next steps will be for the patients,” says Hugo De Oliveira, a PhD student in Health Systems Engineering whose CIFRE thesis is funded by HEVA, a company based in Lyon.
Anticipating how a disease will progress and treatment steps helps limit risks to which the patient is exposed. For people with diabetes — the example used by the researchers in their work — the process is based on detecting weak signals that are precursors of complications as early as possible. For a given patient, the analysis would focus on several years of treatment records and a comparison with other diabetic patients. This would make it possible to determine the patient’s risk of developing renal failure or requiring an amputation related to diabetes.
In order to predict these progressions, the researchers do not rely on personal medical data, such as X-rays or biological analyses. They use medico-administrative data from the national health data system (SNDS). “In 2006, activity-based pricing was put into place,” notes Hugo De Oliveira. With this shift in the principle of funding for healthcare institutions, a large database was created to provide hospitals with the necessary information for reimbursement of treatment. “It’s a very useful database for us, because each line collects information about a patient’s stay: age, sex, care received, primary diagnosis, associated pathologies from which they suffer etc,” says the young researcher.
An entire pathway in one graph
Vincent Augusto’s team is developing algorithms that analyze these large volumes of data. Patients are sorted and put into groups with similar criteria. Different care pathway categories can then be established, each of which groups together several thousands of similar pathways (similar patients, identical complications etc.). In one category — diabetic patients who have undergone amputation for example — the algorithm analyzes all of the steps for the entire group of patients in order to deduce which ones are most characteristic. A graph is produced to represent the typical pathway for this category of patient. It may then be used as a reference to find out whether a patient in the early stages of the disease is following similar steps, and to determine the probability that he/she belongs to this category.
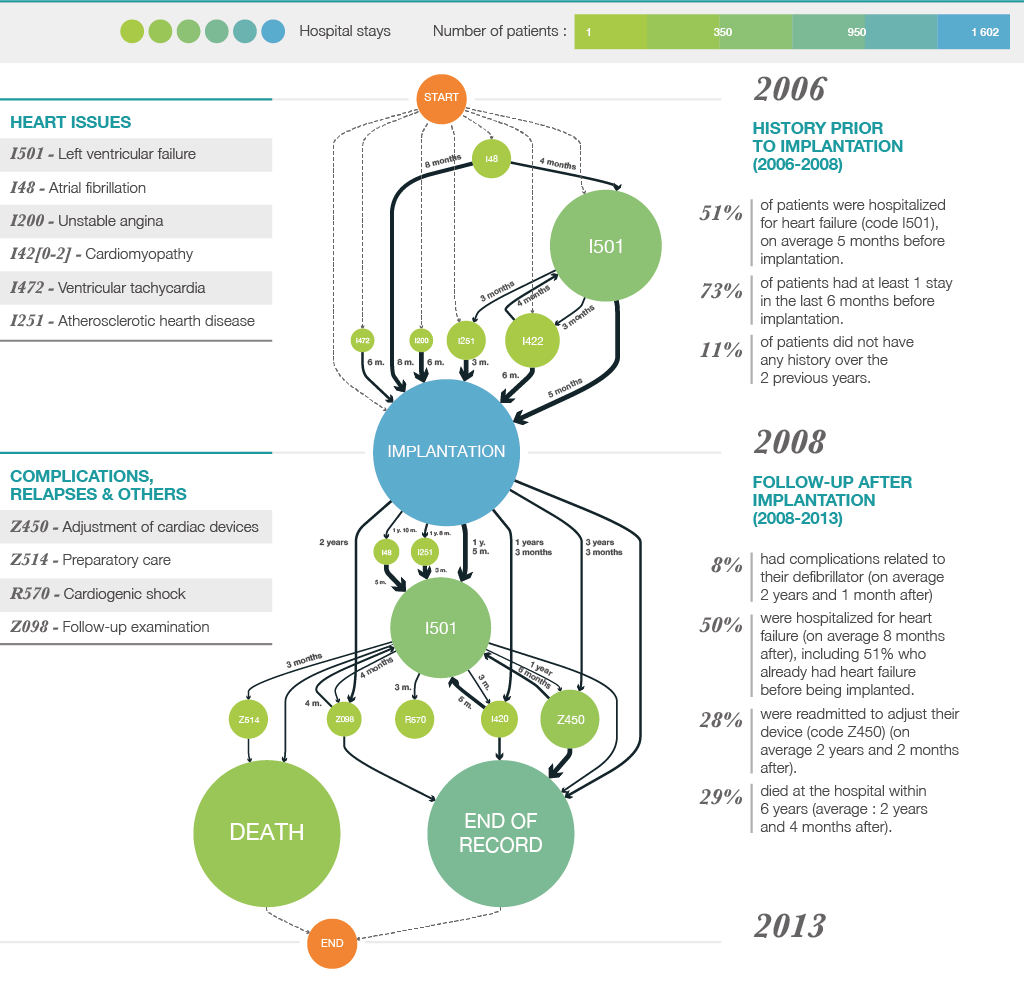
This graph represents the care pathway for patients monitored over an 8-year period who have had a cardiac defibrillator implanted. The part before the implantation can be used to establish statistics for the steps preceding the procedure. The part after the implantation provides information about the future of patients following the implantation.
In this way, the researchers are working on developing longitudinal graphs: each treatment step represents a point on the graph, and the whole graph can be read chronologically: “Doctors can read the graph very easily and determine where the patient is situated in the sequence of steps that characterize his/her pathway,” explains Hugo De Oliveira. The difficulty with this type of data representation comes from its comprehensiveness: “We have to find a way to fit an entire patient pathway into a single line,” says the PhD student. In order to do so, the team chose to use process mining, a data mining and knowledge extraction tool. Machine learning is another such tool.
Process mining helps make care pathway descriptions more effective and easier to read, but it also provides another benefit: it is not a ‘black box’. This characteristic is often encountered in neural network type algorithms. Such algorithms are effective at processing data, but it is impossible to understand the processes that led to the results of the algorithm. Unlike these algorithms, the process mining algorithms used to predict treatment pathways are transparent. “When a patient is characterized by a type of graph, we’re able to understand why by looking at past treatment steps, and studying each graph for the patient’s categories to understand how the algorithm evaluated the pathway,” says Hugo De Oliveira.
Making artificial intelligence applications more transparent is one of the issues brought forth by the working group that produced a French report on AI led by Cédric Villani. The project is also in keeping with the objectives set by the mathematician and member of the French parliament to facilitate AI experimentation for applications, for healthcare in particular. “Our research directly benefits from policies for opening access to health data,” says the PhD student. This access will continue to open up for the researchers, since later on this year they will be able to use the database of the national health insurance cross-scheme system (SNIIRAM): the 1.2 billion healthcare forms contained in the system will be used to improve the algorithms and better identify patient treatment pathways.
Leave a Reply
Want to join the discussion?Feel free to contribute!