Facial morphology analysis algorithms for choosing glasses
Everyone who wears glasses knows: finding the best pair for your face can be a daunting task… The VESPA project, led by researchers at IMT Lille Douai and hosted by the Teralab platform, is working with Cape Trylive to develop machine learning algorithms that can determine users’ facial characteristics during virtual online fittings and recommend products based on their morphology.
Do you have a round face? Then the best glasses for you would be angular and narrow designs. Do you have a square face? Then try butterfly-shaped glasses! Based on your face shape, certain types of frames will look better on you than others. For a few years now, plug-ins on opticians’ websites have allowed users to try on glasses virtually. However, they do not offer features to help users find the best frames for their face.
The VESPA project, led by Jacques Boonaert and Stéphane Lecoeuche at the IMT Lille Douai Computer and Control Sciences Research Unit, in collaboration with Pascal Mobuchon of Acep Trylive, a virtual fitting plug-in supplier, aims to develop a machine learning algorithm capable of identifying users’ face shapes and recommending glasses based on their morphological characteristics. “Acep Trylive has developed technology for establishing the key points on users’ faces while they are filmed via webcam during virtual fittings,” Stéphane Lecoeuche explains. “The goal of the VESPA project is to develop algorithms capable of analyzing the position of key elements on faces to determine the user’s morphology.” All the data used in the context of this project are anonymized and hosted on the secure, neutral and sovereign Teralab platform. TeraLab also provides researchers with tools for processing these data sets.
Read more on I’MTech: TeraLab, a big data platform with a European vision
Algorithms that can identify facial morphology
The algorithms developed by the researchers follow a supervised learning logic. “This means that we submit a set of images labeled by human experts to the algorithms, which determine if the person’s face is round, square, oval…” Jacques Boonaert explains.
In addition, the key points that Acep Trylive’s software automatically establishes on the user’s face provide the algorithm with a set of descriptors. For example, a descriptor could be a measurement of the key points on the face: this could be the height of the forehead, the shape of the chin, the width of the face or the jaw, etc. Based on the data labelled by human experts, the algorithm will determine which descriptors are most relevant in recognizing the user’s morphology. “We have tested sub-sets of descriptors. In all, there are over 20,” says Stéphane Lecoeuche. “The algorithms then ascertain the influence of the descriptors on their own to define the best morphological characterization.”
There are three of these morphological classification algorithms. One focuses on the shape of the jaw, the second on the face shape, and the third on the width of the forehead. Digital descriptors of the user’s hair, eye and skin color are also used to propose the most suitable colors for the frames. All of this data is then merged to create recommendations for glasses adapted to the user based on these characteristics.
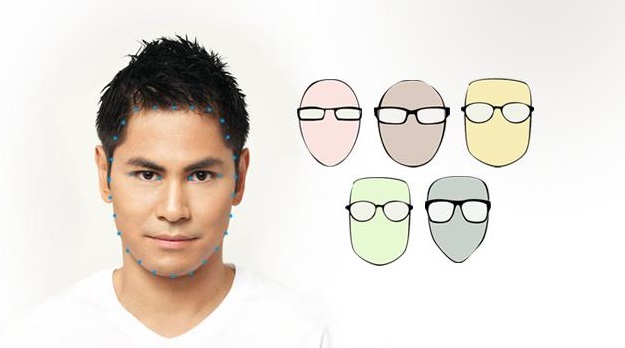
The Acep Trylive software highlights the key points on users’ faces (in blue)
Recommending products based on users’ morphology
Based on the monitoring of behavior and consumers’ history, the researchers were able to determine which glasses each internet user preferred: “Someone tries on a first pair of glasses, then a second, comes back to the first, tries a third pair, then again comes back to the first… In observing this sequence, we can determine which product the person prefers!” Jacques Boonaert explains.
The learning algorithm takes this fitting history into account and statistically consolidates the glasses the user preferred by morphology type. “Thanks to the data from thousands or even hundreds of thousands of fitting sessions, the algorithm makes the connection between the face shape and the products that were tried on,” says Stéphane Lecoeuche. Therefore, for each new user who tries the application, the morphological analysis algorithms will determine their face shape and, depending on the choices of users with a similar morphology, and the recommendation engine will propose a set of products most likely to please them.
Seeking a partner company for further development
“We also had to work on the product’s geometric classification. The problem is that we have not been able to access the data from opticians’ catalogs, which classify glasses by shape, style, color…” Stéphane Lecoeuche explains. The researchers had planned to work on the association between the customer’s morphological characteristics and the products’ geometrical characteristics. While the algorithmic analysis of the facial morphology provided good results, the lack of data on the frames has limited their objectives.
“The other difficulty is that we do not have access to users’ final purchase decisions,” Jacques Boonaert adds. “We are aware that this is sensitive data for businesses. This is why we would like to create a strong partnership with an optician in order to further develop this project.” The researchers wish to implement a second phase of experimentation with a partner company, in which the algorithm could integrate the users’ buying decision and the products’ geometric classification. In the meantime, while waiting to find a company that would like to integrate the project consortium, the research team is continuing its work in machine learning geared towards industry and trade.
Leave a Reply
Want to join the discussion?Feel free to contribute!